

Evaluation
Competitor submissions will be evaluated across four objective areas:
Innovation (25%)
- Originality of Concept: Does the solution introduce a novel approach to addressing social justice issues, or provide a fresh perspective on existing challenges?
- Creativity in Data Use: How creatively does the team use data to uncover insights or address problems in areas like healthcare, education, housing, or employment?
- Integration of Social Justice Values: Does the solution clearly integrate core social justice principles (access, equity, participation, diversity, human rights) into its design and goals?
- Problem-Solving: Does the project provide an innovative way to reduce inequality or improve fairness, especially for marginalized or underserved communities?
Feasibility (25%)
- Technical Viability: Is the solution technically sound and realistically achievable given current technologies and resources?
- Data Availability and Quality: Has the team identified and used reliable, relevant datasets, and are their data choices appropriate for the problem they are tackling?
- Practical Implementation: Can the solution be realistically implemented and scaled in the real world (e.g., by institutions, communities, or governments)?
- Resource Constraints: Does the solution take into account constraints such as budget, time, or access to technology in underserved areas?
Impact (25%)
- Addressing Inequality: How effectively does the solution address inequalities in key areas like education, healthcare, housing, or employment?
- Potential for Societal Change: Does the solution have the potential to create significant, positive social change? Does it align with long-term goals of promoting inclusivity and fairness?
- Sustainability of Impact: Can the solution sustain its impact over time, or does it offer a one-time fix? Does it enable ongoing participation from marginalized groups?
- Measurability: How clearly can the impact of the solution be measured using data-driven metrics, particularly in the context of reducing disparities or improving fairness?
AI Adoption (25%)
- Use of AI/ML Techniques: How effectively does the project leverage AI or machine learning to drive insights, enhance fairness, or improve decision-making in the context of social justice?
- Ethical AI Considerations: Has the team considered the ethical implications of their AI use, particularly with respect to minimizing bias, ensuring fairness, and improving accessibility?
- Transparency and Explainability: Are the AI models transparent and explainable? Does the team consider how users (especially non-experts) will understand or trust the technology?
- Bias Mitigation: How well has the team addressed or mitigated biases in their AI models, especially those that might perpetuate existing inequalities?
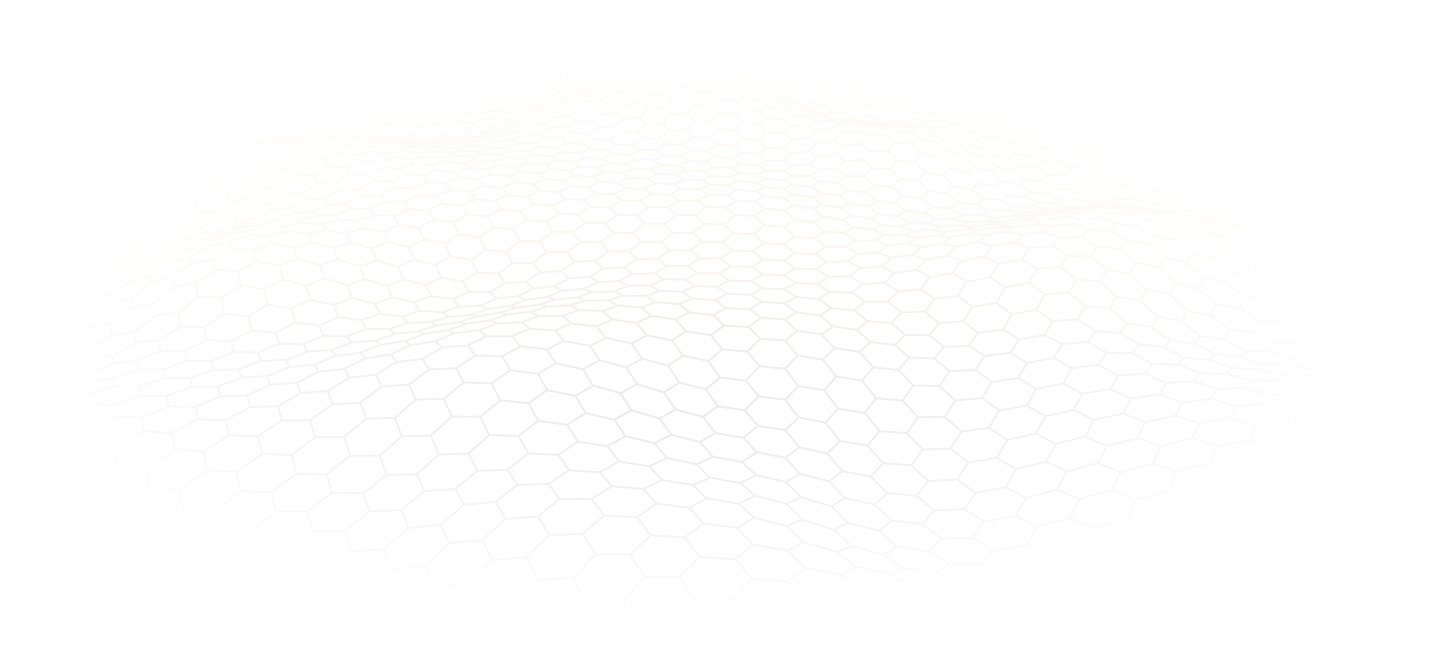
